mirror of
https://github.com/sockspls/badfish
synced 2025-05-07 03:39:35 +00:00
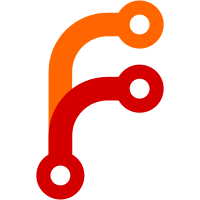
This patch improves the codegen in the AffineTransform::forward function for architectures >=SSSE3. Current code works directly on memory and the compiler cannot see that the stores through outptr do not alias the loads through weights and input32. The solution implemented is to perform the affine transform with local variables as accumulators and only store the result to memory at the end. The number of accumulators required is OutputDimensions / OutputSimdWidth, which means that for the 1024->16 affine transform it requires 4 registers with SSSE3, 2 with AVX2, 1 with AVX512. It also cuts the number of stores required by NumRegs * 256 for each node evaluated. The local accumulators are expected to be assigned to registers, but even if this cannot be done in some case due to register pressure it will help the compiler to see that there is no aliasing between the loads and stores and may still result in better codegen. See https://godbolt.org/z/59aTKbbYc for codegen comparison. passed STC: LLR: 2.94 (-2.94,2.94) <-0.50,2.50> Total: 140328 W: 10635 L: 10358 D: 119335 Ptnml(0-2): 302, 8339, 52636, 8554, 333 closes https://github.com/official-stockfish/Stockfish/pull/3634 No functional change
443 lines
18 KiB
C++
443 lines
18 KiB
C++
/*
|
|
Stockfish, a UCI chess playing engine derived from Glaurung 2.1
|
|
Copyright (C) 2004-2021 The Stockfish developers (see AUTHORS file)
|
|
|
|
Stockfish is free software: you can redistribute it and/or modify
|
|
it under the terms of the GNU General Public License as published by
|
|
the Free Software Foundation, either version 3 of the License, or
|
|
(at your option) any later version.
|
|
|
|
Stockfish is distributed in the hope that it will be useful,
|
|
but WITHOUT ANY WARRANTY; without even the implied warranty of
|
|
MERCHANTABILITY or FITNESS FOR A PARTICULAR PURPOSE. See the
|
|
GNU General Public License for more details.
|
|
|
|
You should have received a copy of the GNU General Public License
|
|
along with this program. If not, see <http://www.gnu.org/licenses/>.
|
|
*/
|
|
|
|
// Definition of layer AffineTransform of NNUE evaluation function
|
|
|
|
#ifndef NNUE_LAYERS_AFFINE_TRANSFORM_H_INCLUDED
|
|
#define NNUE_LAYERS_AFFINE_TRANSFORM_H_INCLUDED
|
|
|
|
#include <iostream>
|
|
#include "../nnue_common.h"
|
|
|
|
namespace Stockfish::Eval::NNUE::Layers {
|
|
|
|
// Affine transformation layer
|
|
template <typename PreviousLayer, IndexType OutDims>
|
|
class AffineTransform {
|
|
public:
|
|
// Input/output type
|
|
using InputType = typename PreviousLayer::OutputType;
|
|
using OutputType = std::int32_t;
|
|
static_assert(std::is_same<InputType, std::uint8_t>::value, "");
|
|
|
|
// Number of input/output dimensions
|
|
static constexpr IndexType InputDimensions =
|
|
PreviousLayer::OutputDimensions;
|
|
static constexpr IndexType OutputDimensions = OutDims;
|
|
static constexpr IndexType PaddedInputDimensions =
|
|
ceil_to_multiple<IndexType>(InputDimensions, MaxSimdWidth);
|
|
#if defined (USE_AVX512)
|
|
static constexpr const IndexType OutputSimdWidth = SimdWidth / 2;
|
|
#elif defined (USE_SSSE3)
|
|
static constexpr const IndexType OutputSimdWidth = SimdWidth / 4;
|
|
#endif
|
|
|
|
// Size of forward propagation buffer used in this layer
|
|
static constexpr std::size_t SelfBufferSize =
|
|
ceil_to_multiple(OutputDimensions * sizeof(OutputType), CacheLineSize);
|
|
|
|
// Size of the forward propagation buffer used from the input layer to this layer
|
|
static constexpr std::size_t BufferSize =
|
|
PreviousLayer::BufferSize + SelfBufferSize;
|
|
|
|
// Hash value embedded in the evaluation file
|
|
static constexpr std::uint32_t get_hash_value() {
|
|
std::uint32_t hashValue = 0xCC03DAE4u;
|
|
hashValue += OutputDimensions;
|
|
hashValue ^= PreviousLayer::get_hash_value() >> 1;
|
|
hashValue ^= PreviousLayer::get_hash_value() << 31;
|
|
return hashValue;
|
|
}
|
|
|
|
// Read network parameters
|
|
bool read_parameters(std::istream& stream) {
|
|
if (!previousLayer.read_parameters(stream)) return false;
|
|
for (std::size_t i = 0; i < OutputDimensions; ++i)
|
|
biases[i] = read_little_endian<BiasType>(stream);
|
|
for (std::size_t i = 0; i < OutputDimensions * PaddedInputDimensions; ++i)
|
|
#if !defined (USE_SSSE3)
|
|
weights[i] = read_little_endian<WeightType>(stream);
|
|
#else
|
|
weights[
|
|
(i / 4) % (PaddedInputDimensions / 4) * OutputDimensions * 4 +
|
|
i / PaddedInputDimensions * 4 +
|
|
i % 4
|
|
] = read_little_endian<WeightType>(stream);
|
|
#endif
|
|
|
|
return !stream.fail();
|
|
}
|
|
|
|
// Write network parameters
|
|
bool write_parameters(std::ostream& stream) const {
|
|
if (!previousLayer.write_parameters(stream)) return false;
|
|
for (std::size_t i = 0; i < OutputDimensions; ++i)
|
|
write_little_endian<BiasType>(stream, biases[i]);
|
|
#if !defined (USE_SSSE3)
|
|
for (std::size_t i = 0; i < OutputDimensions * PaddedInputDimensions; ++i)
|
|
write_little_endian<WeightType>(stream, weights[i]);
|
|
#else
|
|
std::unique_ptr<WeightType[]> unscrambledWeights = std::make_unique<WeightType[]>(OutputDimensions * PaddedInputDimensions);
|
|
for (std::size_t i = 0; i < OutputDimensions * PaddedInputDimensions; ++i) {
|
|
unscrambledWeights[i] =
|
|
weights[
|
|
(i / 4) % (PaddedInputDimensions / 4) * OutputDimensions * 4 +
|
|
i / PaddedInputDimensions * 4 +
|
|
i % 4
|
|
];
|
|
}
|
|
|
|
for (std::size_t i = 0; i < OutputDimensions * PaddedInputDimensions; ++i)
|
|
write_little_endian<WeightType>(stream, unscrambledWeights[i]);
|
|
#endif
|
|
|
|
return !stream.fail();
|
|
}
|
|
|
|
// Forward propagation
|
|
const OutputType* propagate(
|
|
const TransformedFeatureType* transformedFeatures, char* buffer) const {
|
|
const auto input = previousLayer.propagate(
|
|
transformedFeatures, buffer + SelfBufferSize);
|
|
|
|
#if defined (USE_AVX512)
|
|
|
|
[[maybe_unused]] const __m512i Ones512 = _mm512_set1_epi16(1);
|
|
|
|
[[maybe_unused]] auto m512_hadd = [](__m512i sum, int bias) -> int {
|
|
return _mm512_reduce_add_epi32(sum) + bias;
|
|
};
|
|
|
|
[[maybe_unused]] auto m512_add_dpbusd_epi32 = [=](__m512i& acc, __m512i a, __m512i b) {
|
|
#if defined (USE_VNNI)
|
|
acc = _mm512_dpbusd_epi32(acc, a, b);
|
|
#else
|
|
__m512i product0 = _mm512_maddubs_epi16(a, b);
|
|
product0 = _mm512_madd_epi16(product0, Ones512);
|
|
acc = _mm512_add_epi32(acc, product0);
|
|
#endif
|
|
};
|
|
|
|
[[maybe_unused]] auto m512_add_dpbusd_epi32x4 = [=](__m512i& acc, __m512i a0, __m512i b0, __m512i a1, __m512i b1,
|
|
__m512i a2, __m512i b2, __m512i a3, __m512i b3) {
|
|
#if defined (USE_VNNI)
|
|
acc = _mm512_dpbusd_epi32(acc, a0, b0);
|
|
acc = _mm512_dpbusd_epi32(acc, a1, b1);
|
|
acc = _mm512_dpbusd_epi32(acc, a2, b2);
|
|
acc = _mm512_dpbusd_epi32(acc, a3, b3);
|
|
#else
|
|
__m512i product0 = _mm512_maddubs_epi16(a0, b0);
|
|
__m512i product1 = _mm512_maddubs_epi16(a1, b1);
|
|
__m512i product2 = _mm512_maddubs_epi16(a2, b2);
|
|
__m512i product3 = _mm512_maddubs_epi16(a3, b3);
|
|
product0 = _mm512_adds_epi16(product0, product1);
|
|
product0 = _mm512_madd_epi16(product0, Ones512);
|
|
product2 = _mm512_adds_epi16(product2, product3);
|
|
product2 = _mm512_madd_epi16(product2, Ones512);
|
|
acc = _mm512_add_epi32(acc, _mm512_add_epi32(product0, product2));
|
|
#endif
|
|
};
|
|
|
|
#endif
|
|
#if defined (USE_AVX2)
|
|
|
|
[[maybe_unused]] const __m256i Ones256 = _mm256_set1_epi16(1);
|
|
|
|
[[maybe_unused]] auto m256_hadd = [](__m256i sum, int bias) -> int {
|
|
__m128i sum128 = _mm_add_epi32(_mm256_castsi256_si128(sum), _mm256_extracti128_si256(sum, 1));
|
|
sum128 = _mm_add_epi32(sum128, _mm_shuffle_epi32(sum128, _MM_PERM_BADC));
|
|
sum128 = _mm_add_epi32(sum128, _mm_shuffle_epi32(sum128, _MM_PERM_CDAB));
|
|
return _mm_cvtsi128_si32(sum128) + bias;
|
|
};
|
|
|
|
[[maybe_unused]] auto m256_add_dpbusd_epi32 = [=](__m256i& acc, __m256i a, __m256i b) {
|
|
#if defined (USE_VNNI)
|
|
acc = _mm256_dpbusd_epi32(acc, a, b);
|
|
#else
|
|
__m256i product0 = _mm256_maddubs_epi16(a, b);
|
|
product0 = _mm256_madd_epi16(product0, Ones256);
|
|
acc = _mm256_add_epi32(acc, product0);
|
|
#endif
|
|
};
|
|
|
|
[[maybe_unused]] auto m256_add_dpbusd_epi32x4 = [=](__m256i& acc, __m256i a0, __m256i b0, __m256i a1, __m256i b1,
|
|
__m256i a2, __m256i b2, __m256i a3, __m256i b3) {
|
|
#if defined (USE_VNNI)
|
|
acc = _mm256_dpbusd_epi32(acc, a0, b0);
|
|
acc = _mm256_dpbusd_epi32(acc, a1, b1);
|
|
acc = _mm256_dpbusd_epi32(acc, a2, b2);
|
|
acc = _mm256_dpbusd_epi32(acc, a3, b3);
|
|
#else
|
|
__m256i product0 = _mm256_maddubs_epi16(a0, b0);
|
|
__m256i product1 = _mm256_maddubs_epi16(a1, b1);
|
|
__m256i product2 = _mm256_maddubs_epi16(a2, b2);
|
|
__m256i product3 = _mm256_maddubs_epi16(a3, b3);
|
|
product0 = _mm256_adds_epi16(product0, product1);
|
|
product0 = _mm256_madd_epi16(product0, Ones256);
|
|
product2 = _mm256_adds_epi16(product2, product3);
|
|
product2 = _mm256_madd_epi16(product2, Ones256);
|
|
acc = _mm256_add_epi32(acc, _mm256_add_epi32(product0, product2));
|
|
#endif
|
|
};
|
|
|
|
#endif
|
|
#if defined (USE_SSSE3)
|
|
|
|
[[maybe_unused]] const __m128i Ones128 = _mm_set1_epi16(1);
|
|
|
|
[[maybe_unused]] auto m128_hadd = [](__m128i sum, int bias) -> int {
|
|
sum = _mm_add_epi32(sum, _mm_shuffle_epi32(sum, 0x4E)); //_MM_PERM_BADC
|
|
sum = _mm_add_epi32(sum, _mm_shuffle_epi32(sum, 0xB1)); //_MM_PERM_CDAB
|
|
return _mm_cvtsi128_si32(sum) + bias;
|
|
};
|
|
|
|
[[maybe_unused]] auto m128_add_dpbusd_epi32 = [=](__m128i& acc, __m128i a, __m128i b) {
|
|
__m128i product0 = _mm_maddubs_epi16(a, b);
|
|
product0 = _mm_madd_epi16(product0, Ones128);
|
|
acc = _mm_add_epi32(acc, product0);
|
|
};
|
|
|
|
[[maybe_unused]] auto m128_add_dpbusd_epi32x4 = [=](__m128i& acc, __m128i a0, __m128i b0, __m128i a1, __m128i b1,
|
|
__m128i a2, __m128i b2, __m128i a3, __m128i b3) {
|
|
__m128i product0 = _mm_maddubs_epi16(a0, b0);
|
|
__m128i product1 = _mm_maddubs_epi16(a1, b1);
|
|
__m128i product2 = _mm_maddubs_epi16(a2, b2);
|
|
__m128i product3 = _mm_maddubs_epi16(a3, b3);
|
|
product0 = _mm_adds_epi16(product0, product1);
|
|
product0 = _mm_madd_epi16(product0, Ones128);
|
|
product2 = _mm_adds_epi16(product2, product3);
|
|
product2 = _mm_madd_epi16(product2, Ones128);
|
|
acc = _mm_add_epi32(acc, _mm_add_epi32(product0, product2));
|
|
};
|
|
|
|
#endif
|
|
|
|
#if defined (USE_AVX512)
|
|
using vec_t = __m512i;
|
|
#define vec_setzero _mm512_setzero_si512
|
|
#define vec_set_32 _mm512_set1_epi32
|
|
auto& vec_add_dpbusd_32 = m512_add_dpbusd_epi32;
|
|
auto& vec_add_dpbusd_32x4 = m512_add_dpbusd_epi32x4;
|
|
auto& vec_hadd = m512_hadd;
|
|
#elif defined (USE_AVX2)
|
|
using vec_t = __m256i;
|
|
#define vec_setzero _mm256_setzero_si256
|
|
#define vec_set_32 _mm256_set1_epi32
|
|
auto& vec_add_dpbusd_32 = m256_add_dpbusd_epi32;
|
|
auto& vec_add_dpbusd_32x4 = m256_add_dpbusd_epi32x4;
|
|
auto& vec_hadd = m256_hadd;
|
|
#elif defined (USE_SSSE3)
|
|
using vec_t = __m128i;
|
|
#define vec_setzero _mm_setzero_si128
|
|
#define vec_set_32 _mm_set1_epi32
|
|
auto& vec_add_dpbusd_32 = m128_add_dpbusd_epi32;
|
|
auto& vec_add_dpbusd_32x4 = m128_add_dpbusd_epi32x4;
|
|
auto& vec_hadd = m128_hadd;
|
|
#endif
|
|
|
|
#if defined (USE_SSSE3)
|
|
const auto output = reinterpret_cast<OutputType*>(buffer);
|
|
const auto inputVector = reinterpret_cast<const vec_t*>(input);
|
|
|
|
static_assert(OutputDimensions % OutputSimdWidth == 0 || OutputDimensions == 1);
|
|
|
|
// OutputDimensions is either 1 or a multiple of SimdWidth
|
|
// because then it is also an input dimension.
|
|
if constexpr (OutputDimensions % OutputSimdWidth == 0)
|
|
{
|
|
static_assert(InputDimensions % 16 == 0);
|
|
|
|
constexpr IndexType NumChunks = InputDimensions / 4;
|
|
constexpr IndexType NumRegs = OutputDimensions / OutputSimdWidth;
|
|
|
|
const auto input32 = reinterpret_cast<const std::int32_t*>(input);
|
|
const vec_t* biasvec = reinterpret_cast<const vec_t*>(biases);
|
|
vec_t outs[NumRegs];
|
|
for (IndexType k = 0; k < NumRegs; ++k)
|
|
outs[k] = biasvec[k];
|
|
|
|
for (IndexType i = 0; i < NumChunks; i += 4)
|
|
{
|
|
const vec_t in0 = vec_set_32(input32[i + 0]);
|
|
const vec_t in1 = vec_set_32(input32[i + 1]);
|
|
const vec_t in2 = vec_set_32(input32[i + 2]);
|
|
const vec_t in3 = vec_set_32(input32[i + 3]);
|
|
const auto col0 = reinterpret_cast<const vec_t*>(&weights[(i + 0) * OutputDimensions * 4]);
|
|
const auto col1 = reinterpret_cast<const vec_t*>(&weights[(i + 1) * OutputDimensions * 4]);
|
|
const auto col2 = reinterpret_cast<const vec_t*>(&weights[(i + 2) * OutputDimensions * 4]);
|
|
const auto col3 = reinterpret_cast<const vec_t*>(&weights[(i + 3) * OutputDimensions * 4]);
|
|
for (IndexType k = 0; k < NumRegs; ++k)
|
|
vec_add_dpbusd_32x4(outs[k], in0, col0[k], in1, col1[k], in2, col2[k], in3, col3[k]);
|
|
}
|
|
|
|
vec_t* outptr = reinterpret_cast<vec_t*>(output);
|
|
for (IndexType k = 0; k < NumRegs; ++k)
|
|
outptr[k] = outs[k];
|
|
}
|
|
else if constexpr (OutputDimensions == 1)
|
|
{
|
|
static_assert(InputDimensions % 4 == 0);
|
|
|
|
#if defined (USE_AVX512)
|
|
if constexpr (PaddedInputDimensions % (SimdWidth * 2) != 0)
|
|
{
|
|
constexpr IndexType NumChunks = PaddedInputDimensions / SimdWidth;
|
|
const auto inputVector256 = reinterpret_cast<const __m256i*>(input);
|
|
|
|
__m256i sum0 = _mm256_setzero_si256();
|
|
const auto row0 = reinterpret_cast<const __m256i*>(&weights[0]);
|
|
|
|
for (int j = 0; j < (int)NumChunks; ++j)
|
|
{
|
|
const __m256i in = inputVector256[j];
|
|
m256_add_dpbusd_epi32(sum0, in, row0[j]);
|
|
}
|
|
output[0] = m256_hadd(sum0, biases[0]);
|
|
}
|
|
else
|
|
#endif
|
|
{
|
|
#if defined (USE_AVX512)
|
|
constexpr IndexType NumChunks = PaddedInputDimensions / (SimdWidth * 2);
|
|
#else
|
|
constexpr IndexType NumChunks = PaddedInputDimensions / SimdWidth;
|
|
#endif
|
|
vec_t sum0 = vec_setzero();
|
|
const auto row0 = reinterpret_cast<const vec_t*>(&weights[0]);
|
|
|
|
for (int j = 0; j < (int)NumChunks; ++j)
|
|
{
|
|
const vec_t in = inputVector[j];
|
|
vec_add_dpbusd_32(sum0, in, row0[j]);
|
|
}
|
|
output[0] = vec_hadd(sum0, biases[0]);
|
|
}
|
|
}
|
|
|
|
#else
|
|
|
|
// Use old implementation for the other architectures.
|
|
|
|
auto output = reinterpret_cast<OutputType*>(buffer);
|
|
|
|
#if defined(USE_SSE2)
|
|
// At least a multiple of 16, with SSE2.
|
|
static_assert(InputDimensions % SimdWidth == 0);
|
|
constexpr IndexType NumChunks = InputDimensions / SimdWidth;
|
|
const __m128i Zeros = _mm_setzero_si128();
|
|
const auto inputVector = reinterpret_cast<const __m128i*>(input);
|
|
|
|
#elif defined(USE_MMX)
|
|
static_assert(InputDimensions % SimdWidth == 0);
|
|
constexpr IndexType NumChunks = InputDimensions / SimdWidth;
|
|
const __m64 Zeros = _mm_setzero_si64();
|
|
const auto inputVector = reinterpret_cast<const __m64*>(input);
|
|
|
|
#elif defined(USE_NEON)
|
|
static_assert(InputDimensions % SimdWidth == 0);
|
|
constexpr IndexType NumChunks = InputDimensions / SimdWidth;
|
|
const auto inputVector = reinterpret_cast<const int8x8_t*>(input);
|
|
#endif
|
|
|
|
for (IndexType i = 0; i < OutputDimensions; ++i) {
|
|
const IndexType offset = i * PaddedInputDimensions;
|
|
|
|
#if defined(USE_SSE2)
|
|
__m128i sumLo = _mm_cvtsi32_si128(biases[i]);
|
|
__m128i sumHi = Zeros;
|
|
const auto row = reinterpret_cast<const __m128i*>(&weights[offset]);
|
|
for (IndexType j = 0; j < NumChunks; ++j) {
|
|
__m128i row_j = _mm_load_si128(&row[j]);
|
|
__m128i input_j = _mm_load_si128(&inputVector[j]);
|
|
__m128i extendedRowLo = _mm_srai_epi16(_mm_unpacklo_epi8(row_j, row_j), 8);
|
|
__m128i extendedRowHi = _mm_srai_epi16(_mm_unpackhi_epi8(row_j, row_j), 8);
|
|
__m128i extendedInputLo = _mm_unpacklo_epi8(input_j, Zeros);
|
|
__m128i extendedInputHi = _mm_unpackhi_epi8(input_j, Zeros);
|
|
__m128i productLo = _mm_madd_epi16(extendedRowLo, extendedInputLo);
|
|
__m128i productHi = _mm_madd_epi16(extendedRowHi, extendedInputHi);
|
|
sumLo = _mm_add_epi32(sumLo, productLo);
|
|
sumHi = _mm_add_epi32(sumHi, productHi);
|
|
}
|
|
__m128i sum = _mm_add_epi32(sumLo, sumHi);
|
|
__m128i sumHigh_64 = _mm_shuffle_epi32(sum, _MM_SHUFFLE(1, 0, 3, 2));
|
|
sum = _mm_add_epi32(sum, sumHigh_64);
|
|
__m128i sum_second_32 = _mm_shufflelo_epi16(sum, _MM_SHUFFLE(1, 0, 3, 2));
|
|
sum = _mm_add_epi32(sum, sum_second_32);
|
|
output[i] = _mm_cvtsi128_si32(sum);
|
|
|
|
#elif defined(USE_MMX)
|
|
__m64 sumLo = _mm_cvtsi32_si64(biases[i]);
|
|
__m64 sumHi = Zeros;
|
|
const auto row = reinterpret_cast<const __m64*>(&weights[offset]);
|
|
for (IndexType j = 0; j < NumChunks; ++j) {
|
|
__m64 row_j = row[j];
|
|
__m64 input_j = inputVector[j];
|
|
__m64 extendedRowLo = _mm_srai_pi16(_mm_unpacklo_pi8(row_j, row_j), 8);
|
|
__m64 extendedRowHi = _mm_srai_pi16(_mm_unpackhi_pi8(row_j, row_j), 8);
|
|
__m64 extendedInputLo = _mm_unpacklo_pi8(input_j, Zeros);
|
|
__m64 extendedInputHi = _mm_unpackhi_pi8(input_j, Zeros);
|
|
__m64 productLo = _mm_madd_pi16(extendedRowLo, extendedInputLo);
|
|
__m64 productHi = _mm_madd_pi16(extendedRowHi, extendedInputHi);
|
|
sumLo = _mm_add_pi32(sumLo, productLo);
|
|
sumHi = _mm_add_pi32(sumHi, productHi);
|
|
}
|
|
__m64 sum = _mm_add_pi32(sumLo, sumHi);
|
|
sum = _mm_add_pi32(sum, _mm_unpackhi_pi32(sum, sum));
|
|
output[i] = _mm_cvtsi64_si32(sum);
|
|
|
|
#elif defined(USE_NEON)
|
|
int32x4_t sum = {biases[i]};
|
|
const auto row = reinterpret_cast<const int8x8_t*>(&weights[offset]);
|
|
for (IndexType j = 0; j < NumChunks; ++j) {
|
|
int16x8_t product = vmull_s8(inputVector[j * 2], row[j * 2]);
|
|
product = vmlal_s8(product, inputVector[j * 2 + 1], row[j * 2 + 1]);
|
|
sum = vpadalq_s16(sum, product);
|
|
}
|
|
output[i] = sum[0] + sum[1] + sum[2] + sum[3];
|
|
|
|
#else
|
|
OutputType sum = biases[i];
|
|
for (IndexType j = 0; j < InputDimensions; ++j) {
|
|
sum += weights[offset + j] * input[j];
|
|
}
|
|
output[i] = sum;
|
|
#endif
|
|
|
|
}
|
|
#if defined(USE_MMX)
|
|
_mm_empty();
|
|
#endif
|
|
|
|
#endif
|
|
|
|
return output;
|
|
}
|
|
|
|
private:
|
|
using BiasType = OutputType;
|
|
using WeightType = std::int8_t;
|
|
|
|
PreviousLayer previousLayer;
|
|
|
|
alignas(CacheLineSize) BiasType biases[OutputDimensions];
|
|
alignas(CacheLineSize) WeightType weights[OutputDimensions * PaddedInputDimensions];
|
|
};
|
|
|
|
} // namespace Stockfish::Eval::NNUE::Layers
|
|
|
|
#endif // #ifndef NNUE_LAYERS_AFFINE_TRANSFORM_H_INCLUDED
|