mirror of
https://github.com/sockspls/badfish
synced 2025-05-02 01:29:36 +00:00
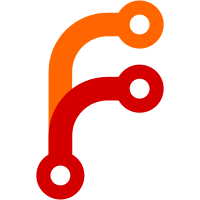
Part 1: PyTorch Training, linrock Trained with a 10-stage sequence from scratch, starting in May 2023: https://github.com/linrock/nnue-tools/blob/master/exp-sequences/3072-10stage-SFNNv9.yml While the training methods were similar to the L1-2560 training sequence, the last two stages introduced min-v2 binpacks, where bestmove capture and in-check position scores were not zeroed during minimization, for compatibility with skipping SEE >= 0 positions and future research. Training data can be found at: https://robotmoon.com/nnue-training-data This net was tested at epoch 679 of the 10th training stage: https://tests.stockfishchess.org/tests/view/65f32e460ec64f0526c48dbc Part 2: SPSA Training, Viren6 The net was then SPSA tuned. This consisted of the output weights (32 * 8) and biases (8) as well as the L3 biases (32 * 8) and L2 biases (16 * 8), totalling 648 params in total. The SPSA tune can be found here: https://tests.stockfishchess.org/tests/view/65fc33ba0ec64f0526c512e3 With the help of Disservin , the initial weights were extracted with: https://github.com/Viren6/Stockfish/tree/new228 The net was saved with the tuned weights using: https://github.com/Viren6/Stockfish/tree/new241 Earlier nets of the SPSA failed STC compared to the base 3072 net of part 1: https://tests.stockfishchess.org/tests/view/65ff356e0ec64f0526c53c98 Therefore it is suspected that the SPSA at VVLTC has added extra scaling on top of the scaling of increasing the L1 size. Passed VVLTC 1: https://tests.stockfishchess.org/tests/view/6604a9020ec64f0526c583da LLR: 2.94 (-2.94,2.94) <0.00,2.00> Total: 53042 W: 13554 L: 13256 D: 26232 Ptnml(0-2): 12, 5147, 15903, 5449, 10 Passed VVLTC 2: https://tests.stockfishchess.org/tests/view/660ad1b60ec64f0526c5dd23 LLR: 2.94 (-2.94,2.94) <0.50,2.50> Total: 17506 W: 4574 L: 4315 D: 8617 Ptnml(0-2): 1, 1567, 5362, 1818, 5 STC Elo estimate: https://tests.stockfishchess.org/tests/view/660b834d01aaec5069f87cb0 Elo: -7.66 ± 3.8 (95%) LOS: 0.0% Total: 9618 W: 2440 L: 2652 D: 4526 Ptnml(0-2): 80, 1281, 2261, 1145, 42 nElo: -13.94 ± 6.9 (95%) PairsRatio: 0.87 closes https://tests.stockfishchess.org/tests/view/660b834d01aaec5069f87cb0 bench 1823302 Co-Authored-By: Linmiao Xu <lin@robotmoon.com>
137 lines
5.8 KiB
C++
137 lines
5.8 KiB
C++
/*
|
|
Stockfish, a UCI chess playing engine derived from Glaurung 2.1
|
|
Copyright (C) 2004-2024 The Stockfish developers (see AUTHORS file)
|
|
|
|
Stockfish is free software: you can redistribute it and/or modify
|
|
it under the terms of the GNU General Public License as published by
|
|
the Free Software Foundation, either version 3 of the License, or
|
|
(at your option) any later version.
|
|
|
|
Stockfish is distributed in the hope that it will be useful,
|
|
but WITHOUT ANY WARRANTY; without even the implied warranty of
|
|
MERCHANTABILITY or FITNESS FOR A PARTICULAR PURPOSE. See the
|
|
GNU General Public License for more details.
|
|
|
|
You should have received a copy of the GNU General Public License
|
|
along with this program. If not, see <http://www.gnu.org/licenses/>.
|
|
*/
|
|
|
|
// Input features and network structure used in NNUE evaluation function
|
|
|
|
#ifndef NNUE_ARCHITECTURE_H_INCLUDED
|
|
#define NNUE_ARCHITECTURE_H_INCLUDED
|
|
|
|
#include <cstdint>
|
|
#include <cstring>
|
|
#include <iosfwd>
|
|
|
|
#include "features/half_ka_v2_hm.h"
|
|
#include "layers/affine_transform.h"
|
|
#include "layers/affine_transform_sparse_input.h"
|
|
#include "layers/clipped_relu.h"
|
|
#include "layers/sqr_clipped_relu.h"
|
|
#include "nnue_common.h"
|
|
|
|
namespace Stockfish::Eval::NNUE {
|
|
|
|
// Input features used in evaluation function
|
|
using FeatureSet = Features::HalfKAv2_hm;
|
|
|
|
// Number of input feature dimensions after conversion
|
|
constexpr IndexType TransformedFeatureDimensionsBig = 3072;
|
|
constexpr int L2Big = 15;
|
|
constexpr int L3Big = 32;
|
|
|
|
constexpr IndexType TransformedFeatureDimensionsSmall = 128;
|
|
constexpr int L2Small = 15;
|
|
constexpr int L3Small = 32;
|
|
|
|
constexpr IndexType PSQTBuckets = 8;
|
|
constexpr IndexType LayerStacks = 8;
|
|
|
|
template<IndexType L1, int L2, int L3>
|
|
struct NetworkArchitecture {
|
|
static constexpr IndexType TransformedFeatureDimensions = L1;
|
|
static constexpr int FC_0_OUTPUTS = L2;
|
|
static constexpr int FC_1_OUTPUTS = L3;
|
|
|
|
Layers::AffineTransformSparseInput<TransformedFeatureDimensions, FC_0_OUTPUTS + 1> fc_0;
|
|
Layers::SqrClippedReLU<FC_0_OUTPUTS + 1> ac_sqr_0;
|
|
Layers::ClippedReLU<FC_0_OUTPUTS + 1> ac_0;
|
|
Layers::AffineTransform<FC_0_OUTPUTS * 2, FC_1_OUTPUTS> fc_1;
|
|
Layers::ClippedReLU<FC_1_OUTPUTS> ac_1;
|
|
Layers::AffineTransform<FC_1_OUTPUTS, 1> fc_2;
|
|
|
|
// Hash value embedded in the evaluation file
|
|
static constexpr std::uint32_t get_hash_value() {
|
|
// input slice hash
|
|
std::uint32_t hashValue = 0xEC42E90Du;
|
|
hashValue ^= TransformedFeatureDimensions * 2;
|
|
|
|
hashValue = decltype(fc_0)::get_hash_value(hashValue);
|
|
hashValue = decltype(ac_0)::get_hash_value(hashValue);
|
|
hashValue = decltype(fc_1)::get_hash_value(hashValue);
|
|
hashValue = decltype(ac_1)::get_hash_value(hashValue);
|
|
hashValue = decltype(fc_2)::get_hash_value(hashValue);
|
|
|
|
return hashValue;
|
|
}
|
|
|
|
// Read network parameters
|
|
bool read_parameters(std::istream& stream) {
|
|
return fc_0.read_parameters(stream) && ac_0.read_parameters(stream)
|
|
&& fc_1.read_parameters(stream) && ac_1.read_parameters(stream)
|
|
&& fc_2.read_parameters(stream);
|
|
}
|
|
|
|
// Write network parameters
|
|
bool write_parameters(std::ostream& stream) const {
|
|
return fc_0.write_parameters(stream) && ac_0.write_parameters(stream)
|
|
&& fc_1.write_parameters(stream) && ac_1.write_parameters(stream)
|
|
&& fc_2.write_parameters(stream);
|
|
}
|
|
|
|
std::int32_t propagate(const TransformedFeatureType* transformedFeatures) {
|
|
struct alignas(CacheLineSize) Buffer {
|
|
alignas(CacheLineSize) typename decltype(fc_0)::OutputBuffer fc_0_out;
|
|
alignas(CacheLineSize) typename decltype(ac_sqr_0)::OutputType
|
|
ac_sqr_0_out[ceil_to_multiple<IndexType>(FC_0_OUTPUTS * 2, 32)];
|
|
alignas(CacheLineSize) typename decltype(ac_0)::OutputBuffer ac_0_out;
|
|
alignas(CacheLineSize) typename decltype(fc_1)::OutputBuffer fc_1_out;
|
|
alignas(CacheLineSize) typename decltype(ac_1)::OutputBuffer ac_1_out;
|
|
alignas(CacheLineSize) typename decltype(fc_2)::OutputBuffer fc_2_out;
|
|
|
|
Buffer() { std::memset(this, 0, sizeof(*this)); }
|
|
};
|
|
|
|
#if defined(__clang__) && (__APPLE__)
|
|
// workaround for a bug reported with xcode 12
|
|
static thread_local auto tlsBuffer = std::make_unique<Buffer>();
|
|
// Access TLS only once, cache result.
|
|
Buffer& buffer = *tlsBuffer;
|
|
#else
|
|
alignas(CacheLineSize) static thread_local Buffer buffer;
|
|
#endif
|
|
|
|
fc_0.propagate(transformedFeatures, buffer.fc_0_out);
|
|
ac_sqr_0.propagate(buffer.fc_0_out, buffer.ac_sqr_0_out);
|
|
ac_0.propagate(buffer.fc_0_out, buffer.ac_0_out);
|
|
std::memcpy(buffer.ac_sqr_0_out + FC_0_OUTPUTS, buffer.ac_0_out,
|
|
FC_0_OUTPUTS * sizeof(typename decltype(ac_0)::OutputType));
|
|
fc_1.propagate(buffer.ac_sqr_0_out, buffer.fc_1_out);
|
|
ac_1.propagate(buffer.fc_1_out, buffer.ac_1_out);
|
|
fc_2.propagate(buffer.ac_1_out, buffer.fc_2_out);
|
|
|
|
// buffer.fc_0_out[FC_0_OUTPUTS] is such that 1.0 is equal to 127*(1<<WeightScaleBits) in
|
|
// quantized form, but we want 1.0 to be equal to 600*OutputScale
|
|
std::int32_t fwdOut =
|
|
(buffer.fc_0_out[FC_0_OUTPUTS]) * (600 * OutputScale) / (127 * (1 << WeightScaleBits));
|
|
std::int32_t outputValue = buffer.fc_2_out[0] + fwdOut;
|
|
|
|
return outputValue;
|
|
}
|
|
};
|
|
|
|
} // namespace Stockfish::Eval::NNUE
|
|
|
|
#endif // #ifndef NNUE_ARCHITECTURE_H_INCLUDED
|