mirror of
https://github.com/sockspls/badfish
synced 2025-04-30 08:43:09 +00:00
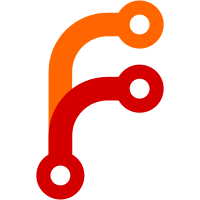
This fixes #3108 and removes some NNUE code that is currently not used. At the moment, do_null_move() copies the accumulator from the previous state into the new state, which is correct. It then clears the "computed_score" flag because the side to move has changed, and with the other side to move NNUE will return a completely different evaluation (normally with changed sign but also with different NNUE-internal tempo bonus). The problem is that do_null_move() clears the wrong flag. It clears the computed_score flag of the old state, not of the new state. It turns out that this almost never affects the search. For example, fixing it does not change the current bench (but it does change the previous bench). This is because the search code usually avoids calling evaluate() after a null move. This PR corrects do_null_move() by removing the computed_score flag altogether. The flag is not needed because nnue_evaluate() is never called twice on a position. This PR also removes some unnecessary {}s and inserts a few blank lines in the modified NNUE files in line with SF coding style. Resulf ot STC non-regression test: LLR: 2.95 (-2.94,2.94) {-1.25,0.25} Total: 26328 W: 3118 L: 3012 D: 20198 Ptnml(0-2): 126, 2208, 8397, 2300, 133 https://tests.stockfishchess.org/tests/view/5f553ccc2d02727c56b36db1 closes https://github.com/official-stockfish/Stockfish/pull/3109 bench: 4109324
370 lines
14 KiB
C++
370 lines
14 KiB
C++
/*
|
|
Stockfish, a UCI chess playing engine derived from Glaurung 2.1
|
|
Copyright (C) 2004-2020 The Stockfish developers (see AUTHORS file)
|
|
|
|
Stockfish is free software: you can redistribute it and/or modify
|
|
it under the terms of the GNU General Public License as published by
|
|
the Free Software Foundation, either version 3 of the License, or
|
|
(at your option) any later version.
|
|
|
|
Stockfish is distributed in the hope that it will be useful,
|
|
but WITHOUT ANY WARRANTY; without even the implied warranty of
|
|
MERCHANTABILITY or FITNESS FOR A PARTICULAR PURPOSE. See the
|
|
GNU General Public License for more details.
|
|
|
|
You should have received a copy of the GNU General Public License
|
|
along with this program. If not, see <http://www.gnu.org/licenses/>.
|
|
*/
|
|
|
|
// A class that converts the input features of the NNUE evaluation function
|
|
|
|
#ifndef NNUE_FEATURE_TRANSFORMER_H_INCLUDED
|
|
#define NNUE_FEATURE_TRANSFORMER_H_INCLUDED
|
|
|
|
#include "nnue_common.h"
|
|
#include "nnue_architecture.h"
|
|
#include "features/index_list.h"
|
|
|
|
#include <cstring> // std::memset()
|
|
|
|
namespace Eval::NNUE {
|
|
|
|
// Input feature converter
|
|
class FeatureTransformer {
|
|
|
|
private:
|
|
// Number of output dimensions for one side
|
|
static constexpr IndexType kHalfDimensions = kTransformedFeatureDimensions;
|
|
|
|
public:
|
|
// Output type
|
|
using OutputType = TransformedFeatureType;
|
|
|
|
// Number of input/output dimensions
|
|
static constexpr IndexType kInputDimensions = RawFeatures::kDimensions;
|
|
static constexpr IndexType kOutputDimensions = kHalfDimensions * 2;
|
|
|
|
// Size of forward propagation buffer
|
|
static constexpr std::size_t kBufferSize =
|
|
kOutputDimensions * sizeof(OutputType);
|
|
|
|
// Hash value embedded in the evaluation file
|
|
static constexpr std::uint32_t GetHashValue() {
|
|
|
|
return RawFeatures::kHashValue ^ kOutputDimensions;
|
|
}
|
|
|
|
// Read network parameters
|
|
bool ReadParameters(std::istream& stream) {
|
|
|
|
for (std::size_t i = 0; i < kHalfDimensions; ++i)
|
|
biases_[i] = read_little_endian<BiasType>(stream);
|
|
for (std::size_t i = 0; i < kHalfDimensions * kInputDimensions; ++i)
|
|
weights_[i] = read_little_endian<WeightType>(stream);
|
|
return !stream.fail();
|
|
}
|
|
|
|
// Proceed with the difference calculation if possible
|
|
bool UpdateAccumulatorIfPossible(const Position& pos) const {
|
|
|
|
const auto now = pos.state();
|
|
if (now->accumulator.computed_accumulation)
|
|
return true;
|
|
|
|
const auto prev = now->previous;
|
|
if (prev && prev->accumulator.computed_accumulation) {
|
|
UpdateAccumulator(pos);
|
|
return true;
|
|
}
|
|
|
|
return false;
|
|
}
|
|
|
|
// Convert input features
|
|
void Transform(const Position& pos, OutputType* output) const {
|
|
|
|
if (!UpdateAccumulatorIfPossible(pos))
|
|
RefreshAccumulator(pos);
|
|
|
|
const auto& accumulation = pos.state()->accumulator.accumulation;
|
|
|
|
#if defined(USE_AVX2)
|
|
constexpr IndexType kNumChunks = kHalfDimensions / kSimdWidth;
|
|
constexpr int kControl = 0b11011000;
|
|
const __m256i kZero = _mm256_setzero_si256();
|
|
|
|
#elif defined(USE_SSE2)
|
|
constexpr IndexType kNumChunks = kHalfDimensions / kSimdWidth;
|
|
|
|
#ifdef USE_SSE41
|
|
const __m128i kZero = _mm_setzero_si128();
|
|
#else
|
|
const __m128i k0x80s = _mm_set1_epi8(-128);
|
|
#endif
|
|
|
|
#elif defined(USE_MMX)
|
|
constexpr IndexType kNumChunks = kHalfDimensions / kSimdWidth;
|
|
const __m64 k0x80s = _mm_set1_pi8(-128);
|
|
|
|
#elif defined(USE_NEON)
|
|
constexpr IndexType kNumChunks = kHalfDimensions / (kSimdWidth / 2);
|
|
const int8x8_t kZero = {0};
|
|
#endif
|
|
|
|
const Color perspectives[2] = {pos.side_to_move(), ~pos.side_to_move()};
|
|
for (IndexType p = 0; p < 2; ++p) {
|
|
const IndexType offset = kHalfDimensions * p;
|
|
|
|
#if defined(USE_AVX2)
|
|
auto out = reinterpret_cast<__m256i*>(&output[offset]);
|
|
for (IndexType j = 0; j < kNumChunks; ++j) {
|
|
__m256i sum0 = _mm256_loadA_si256(
|
|
&reinterpret_cast<const __m256i*>(accumulation[perspectives[p]][0])[j * 2 + 0]);
|
|
__m256i sum1 = _mm256_loadA_si256(
|
|
&reinterpret_cast<const __m256i*>(accumulation[perspectives[p]][0])[j * 2 + 1]);
|
|
_mm256_storeA_si256(&out[j], _mm256_permute4x64_epi64(_mm256_max_epi8(
|
|
_mm256_packs_epi16(sum0, sum1), kZero), kControl));
|
|
}
|
|
|
|
#elif defined(USE_SSE2)
|
|
auto out = reinterpret_cast<__m128i*>(&output[offset]);
|
|
for (IndexType j = 0; j < kNumChunks; ++j) {
|
|
__m128i sum0 = _mm_load_si128(&reinterpret_cast<const __m128i*>(
|
|
accumulation[perspectives[p]][0])[j * 2 + 0]);
|
|
__m128i sum1 = _mm_load_si128(&reinterpret_cast<const __m128i*>(
|
|
accumulation[perspectives[p]][0])[j * 2 + 1]);
|
|
const __m128i packedbytes = _mm_packs_epi16(sum0, sum1);
|
|
|
|
_mm_store_si128(&out[j],
|
|
|
|
#ifdef USE_SSE41
|
|
_mm_max_epi8(packedbytes, kZero)
|
|
#else
|
|
_mm_subs_epi8(_mm_adds_epi8(packedbytes, k0x80s), k0x80s)
|
|
#endif
|
|
|
|
);
|
|
}
|
|
|
|
#elif defined(USE_MMX)
|
|
auto out = reinterpret_cast<__m64*>(&output[offset]);
|
|
for (IndexType j = 0; j < kNumChunks; ++j) {
|
|
__m64 sum0 = *(&reinterpret_cast<const __m64*>(
|
|
accumulation[perspectives[p]][0])[j * 2 + 0]);
|
|
__m64 sum1 = *(&reinterpret_cast<const __m64*>(
|
|
accumulation[perspectives[p]][0])[j * 2 + 1]);
|
|
const __m64 packedbytes = _mm_packs_pi16(sum0, sum1);
|
|
out[j] = _mm_subs_pi8(_mm_adds_pi8(packedbytes, k0x80s), k0x80s);
|
|
}
|
|
|
|
#elif defined(USE_NEON)
|
|
const auto out = reinterpret_cast<int8x8_t*>(&output[offset]);
|
|
for (IndexType j = 0; j < kNumChunks; ++j) {
|
|
int16x8_t sum = reinterpret_cast<const int16x8_t*>(
|
|
accumulation[perspectives[p]][0])[j];
|
|
out[j] = vmax_s8(vqmovn_s16(sum), kZero);
|
|
}
|
|
|
|
#else
|
|
for (IndexType j = 0; j < kHalfDimensions; ++j) {
|
|
BiasType sum = accumulation[static_cast<int>(perspectives[p])][0][j];
|
|
output[offset + j] = static_cast<OutputType>(
|
|
std::max<int>(0, std::min<int>(127, sum)));
|
|
}
|
|
#endif
|
|
|
|
}
|
|
#if defined(USE_MMX)
|
|
_mm_empty();
|
|
#endif
|
|
}
|
|
|
|
private:
|
|
// Calculate cumulative value without using difference calculation
|
|
void RefreshAccumulator(const Position& pos) const {
|
|
|
|
auto& accumulator = pos.state()->accumulator;
|
|
IndexType i = 0;
|
|
Features::IndexList active_indices[2];
|
|
RawFeatures::AppendActiveIndices(pos, kRefreshTriggers[i],
|
|
active_indices);
|
|
for (Color perspective : { WHITE, BLACK }) {
|
|
std::memcpy(accumulator.accumulation[perspective][i], biases_,
|
|
kHalfDimensions * sizeof(BiasType));
|
|
for (const auto index : active_indices[perspective]) {
|
|
const IndexType offset = kHalfDimensions * index;
|
|
#if defined(USE_AVX512)
|
|
auto accumulation = reinterpret_cast<__m512i*>(
|
|
&accumulator.accumulation[perspective][i][0]);
|
|
auto column = reinterpret_cast<const __m512i*>(&weights_[offset]);
|
|
constexpr IndexType kNumChunks = kHalfDimensions / kSimdWidth;
|
|
for (IndexType j = 0; j < kNumChunks; ++j)
|
|
_mm512_storeA_si512(&accumulation[j], _mm512_add_epi16(_mm512_loadA_si512(&accumulation[j]), column[j]));
|
|
|
|
#elif defined(USE_AVX2)
|
|
auto accumulation = reinterpret_cast<__m256i*>(
|
|
&accumulator.accumulation[perspective][i][0]);
|
|
auto column = reinterpret_cast<const __m256i*>(&weights_[offset]);
|
|
constexpr IndexType kNumChunks = kHalfDimensions / (kSimdWidth / 2);
|
|
for (IndexType j = 0; j < kNumChunks; ++j)
|
|
_mm256_storeA_si256(&accumulation[j], _mm256_add_epi16(_mm256_loadA_si256(&accumulation[j]), column[j]));
|
|
|
|
#elif defined(USE_SSE2)
|
|
auto accumulation = reinterpret_cast<__m128i*>(
|
|
&accumulator.accumulation[perspective][i][0]);
|
|
auto column = reinterpret_cast<const __m128i*>(&weights_[offset]);
|
|
constexpr IndexType kNumChunks = kHalfDimensions / (kSimdWidth / 2);
|
|
for (IndexType j = 0; j < kNumChunks; ++j)
|
|
accumulation[j] = _mm_add_epi16(accumulation[j], column[j]);
|
|
|
|
#elif defined(USE_MMX)
|
|
auto accumulation = reinterpret_cast<__m64*>(
|
|
&accumulator.accumulation[perspective][i][0]);
|
|
auto column = reinterpret_cast<const __m64*>(&weights_[offset]);
|
|
constexpr IndexType kNumChunks = kHalfDimensions / (kSimdWidth / 2);
|
|
for (IndexType j = 0; j < kNumChunks; ++j)
|
|
accumulation[j] = _mm_add_pi16(accumulation[j], column[j]);
|
|
|
|
#elif defined(USE_NEON)
|
|
auto accumulation = reinterpret_cast<int16x8_t*>(
|
|
&accumulator.accumulation[perspective][i][0]);
|
|
auto column = reinterpret_cast<const int16x8_t*>(&weights_[offset]);
|
|
constexpr IndexType kNumChunks = kHalfDimensions / (kSimdWidth / 2);
|
|
for (IndexType j = 0; j < kNumChunks; ++j)
|
|
accumulation[j] = vaddq_s16(accumulation[j], column[j]);
|
|
|
|
#else
|
|
for (IndexType j = 0; j < kHalfDimensions; ++j)
|
|
accumulator.accumulation[perspective][i][j] += weights_[offset + j];
|
|
#endif
|
|
|
|
}
|
|
}
|
|
#if defined(USE_MMX)
|
|
_mm_empty();
|
|
#endif
|
|
|
|
accumulator.computed_accumulation = true;
|
|
}
|
|
|
|
// Calculate cumulative value using difference calculation
|
|
void UpdateAccumulator(const Position& pos) const {
|
|
|
|
const auto prev_accumulator = pos.state()->previous->accumulator;
|
|
auto& accumulator = pos.state()->accumulator;
|
|
IndexType i = 0;
|
|
Features::IndexList removed_indices[2], added_indices[2];
|
|
bool reset[2];
|
|
RawFeatures::AppendChangedIndices(pos, kRefreshTriggers[i],
|
|
removed_indices, added_indices, reset);
|
|
for (Color perspective : { WHITE, BLACK }) {
|
|
|
|
#if defined(USE_AVX2)
|
|
constexpr IndexType kNumChunks = kHalfDimensions / (kSimdWidth / 2);
|
|
auto accumulation = reinterpret_cast<__m256i*>(
|
|
&accumulator.accumulation[perspective][i][0]);
|
|
|
|
#elif defined(USE_SSE2)
|
|
constexpr IndexType kNumChunks = kHalfDimensions / (kSimdWidth / 2);
|
|
auto accumulation = reinterpret_cast<__m128i*>(
|
|
&accumulator.accumulation[perspective][i][0]);
|
|
|
|
#elif defined(USE_MMX)
|
|
constexpr IndexType kNumChunks = kHalfDimensions / (kSimdWidth / 2);
|
|
auto accumulation = reinterpret_cast<__m64*>(
|
|
&accumulator.accumulation[perspective][i][0]);
|
|
|
|
#elif defined(USE_NEON)
|
|
constexpr IndexType kNumChunks = kHalfDimensions / (kSimdWidth / 2);
|
|
auto accumulation = reinterpret_cast<int16x8_t*>(
|
|
&accumulator.accumulation[perspective][i][0]);
|
|
#endif
|
|
|
|
if (reset[perspective]) {
|
|
std::memcpy(accumulator.accumulation[perspective][i], biases_,
|
|
kHalfDimensions * sizeof(BiasType));
|
|
} else {
|
|
std::memcpy(accumulator.accumulation[perspective][i],
|
|
prev_accumulator.accumulation[perspective][i],
|
|
kHalfDimensions * sizeof(BiasType));
|
|
// Difference calculation for the deactivated features
|
|
for (const auto index : removed_indices[perspective]) {
|
|
const IndexType offset = kHalfDimensions * index;
|
|
|
|
#if defined(USE_AVX2)
|
|
auto column = reinterpret_cast<const __m256i*>(&weights_[offset]);
|
|
for (IndexType j = 0; j < kNumChunks; ++j)
|
|
accumulation[j] = _mm256_sub_epi16(accumulation[j], column[j]);
|
|
|
|
#elif defined(USE_SSE2)
|
|
auto column = reinterpret_cast<const __m128i*>(&weights_[offset]);
|
|
for (IndexType j = 0; j < kNumChunks; ++j)
|
|
accumulation[j] = _mm_sub_epi16(accumulation[j], column[j]);
|
|
|
|
#elif defined(USE_MMX)
|
|
auto column = reinterpret_cast<const __m64*>(&weights_[offset]);
|
|
for (IndexType j = 0; j < kNumChunks; ++j)
|
|
accumulation[j] = _mm_sub_pi16(accumulation[j], column[j]);
|
|
|
|
#elif defined(USE_NEON)
|
|
auto column = reinterpret_cast<const int16x8_t*>(&weights_[offset]);
|
|
for (IndexType j = 0; j < kNumChunks; ++j)
|
|
accumulation[j] = vsubq_s16(accumulation[j], column[j]);
|
|
|
|
#else
|
|
for (IndexType j = 0; j < kHalfDimensions; ++j)
|
|
accumulator.accumulation[perspective][i][j] -= weights_[offset + j];
|
|
#endif
|
|
|
|
}
|
|
}
|
|
{ // Difference calculation for the activated features
|
|
for (const auto index : added_indices[perspective]) {
|
|
const IndexType offset = kHalfDimensions * index;
|
|
|
|
#if defined(USE_AVX2)
|
|
auto column = reinterpret_cast<const __m256i*>(&weights_[offset]);
|
|
for (IndexType j = 0; j < kNumChunks; ++j)
|
|
accumulation[j] = _mm256_add_epi16(accumulation[j], column[j]);
|
|
|
|
#elif defined(USE_SSE2)
|
|
auto column = reinterpret_cast<const __m128i*>(&weights_[offset]);
|
|
for (IndexType j = 0; j < kNumChunks; ++j)
|
|
accumulation[j] = _mm_add_epi16(accumulation[j], column[j]);
|
|
|
|
#elif defined(USE_MMX)
|
|
auto column = reinterpret_cast<const __m64*>(&weights_[offset]);
|
|
for (IndexType j = 0; j < kNumChunks; ++j)
|
|
accumulation[j] = _mm_add_pi16(accumulation[j], column[j]);
|
|
|
|
#elif defined(USE_NEON)
|
|
auto column = reinterpret_cast<const int16x8_t*>(&weights_[offset]);
|
|
for (IndexType j = 0; j < kNumChunks; ++j)
|
|
accumulation[j] = vaddq_s16(accumulation[j], column[j]);
|
|
|
|
#else
|
|
for (IndexType j = 0; j < kHalfDimensions; ++j)
|
|
accumulator.accumulation[perspective][i][j] += weights_[offset + j];
|
|
#endif
|
|
|
|
}
|
|
}
|
|
}
|
|
#if defined(USE_MMX)
|
|
_mm_empty();
|
|
#endif
|
|
|
|
accumulator.computed_accumulation = true;
|
|
}
|
|
|
|
using BiasType = std::int16_t;
|
|
using WeightType = std::int16_t;
|
|
|
|
alignas(kCacheLineSize) BiasType biases_[kHalfDimensions];
|
|
alignas(kCacheLineSize)
|
|
WeightType weights_[kHalfDimensions * kInputDimensions];
|
|
};
|
|
|
|
} // namespace Eval::NNUE
|
|
|
|
#endif // #ifndef NNUE_FEATURE_TRANSFORMER_H_INCLUDED
|